Fine-scale maps of recreational use
Data on recreational use are critical for evaluating proposed policies and management options for public lands. On-site counts and intercept survey programs provide invaluable information on the amount and character of recreation use on public lands, but they are limited by the time and expense required to survey visitors. Additionally, the timeline to inform policy and management is often too short to wait for new data collection.
To aid governments and community organizations who see increasing and varying demand for outdoor recreation, we are innovating the use of crowd-sourced data and volunteered information from social media users and citizen scientists as fertile data on recreation. The Outdoor R&D team researches ways to mix big digital data streams with traditional data sources in order to map and measure public land use more efficiently, and at finer spatial and temporal scales. We have shown through years of research in diverse settings worldwide that the locations of photographs that are uploaded to photo-sharing websites such as Flickr and Instagram are reliable sources of data on the numbers of visitors, and these methods can be scaled up to study many destinations and points in time.
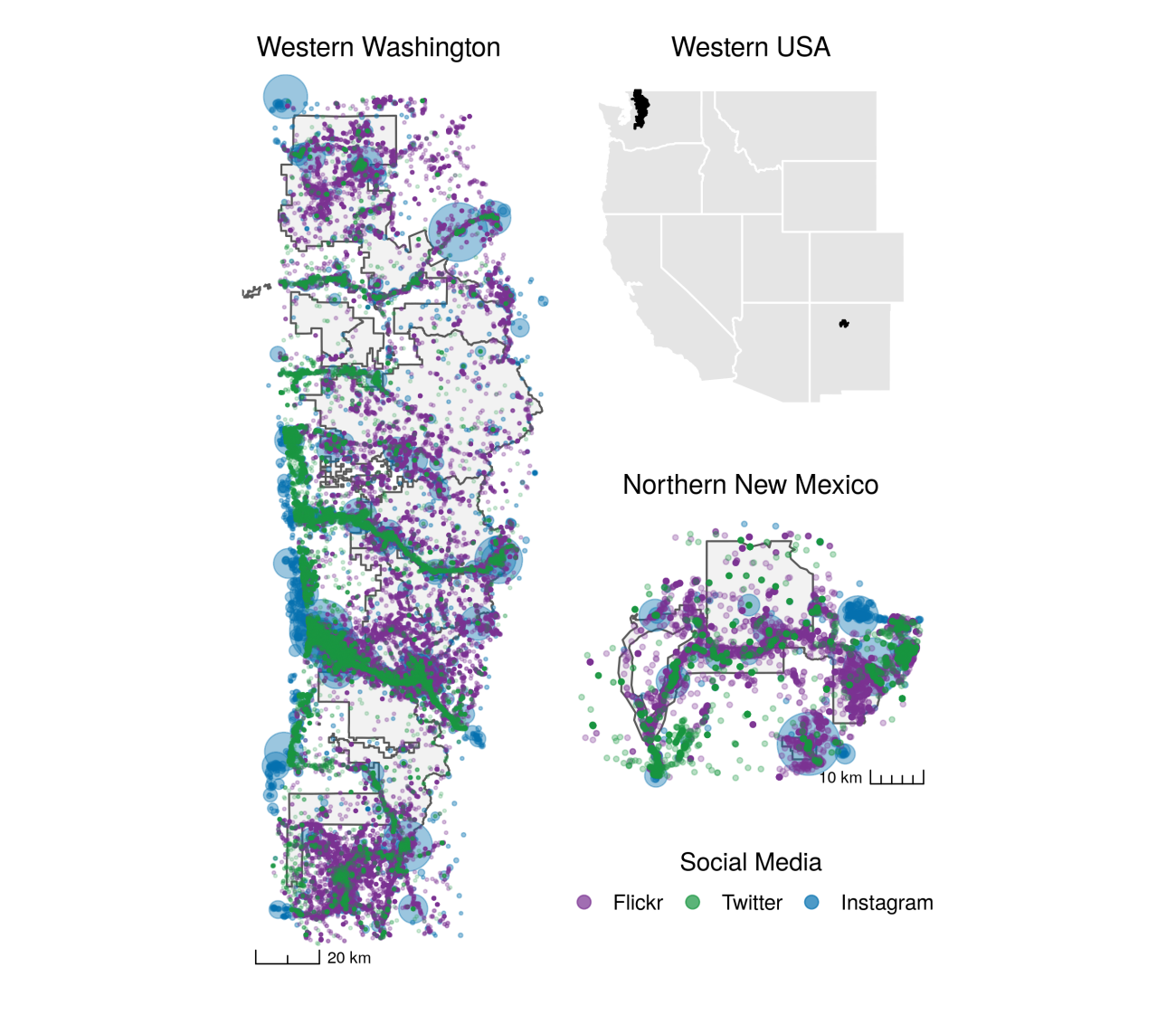
Across a wide range of sites and locations around the world, we find that the density of social media posts is typically 60-90% correlated with recorded use. In National Forests, for example, the density of photographs taken by hikers on trails is correlated in space and time with on-site counts of visitors and with trip reports posted to online hiking guides. Similarly, at National Parks in U.S., seasonal trends in recreational use are mirrored by seasonal trends in the popularity of parks online. In New York City and the Twin Cities, trends in urban park use are mirrored by trends in the numbers and types of social media postings from the same locations. Currently we are developing new statistical models for predicting visitor use with higher accuracy in space and time – calibrated using a relatively small number of on-site counts.
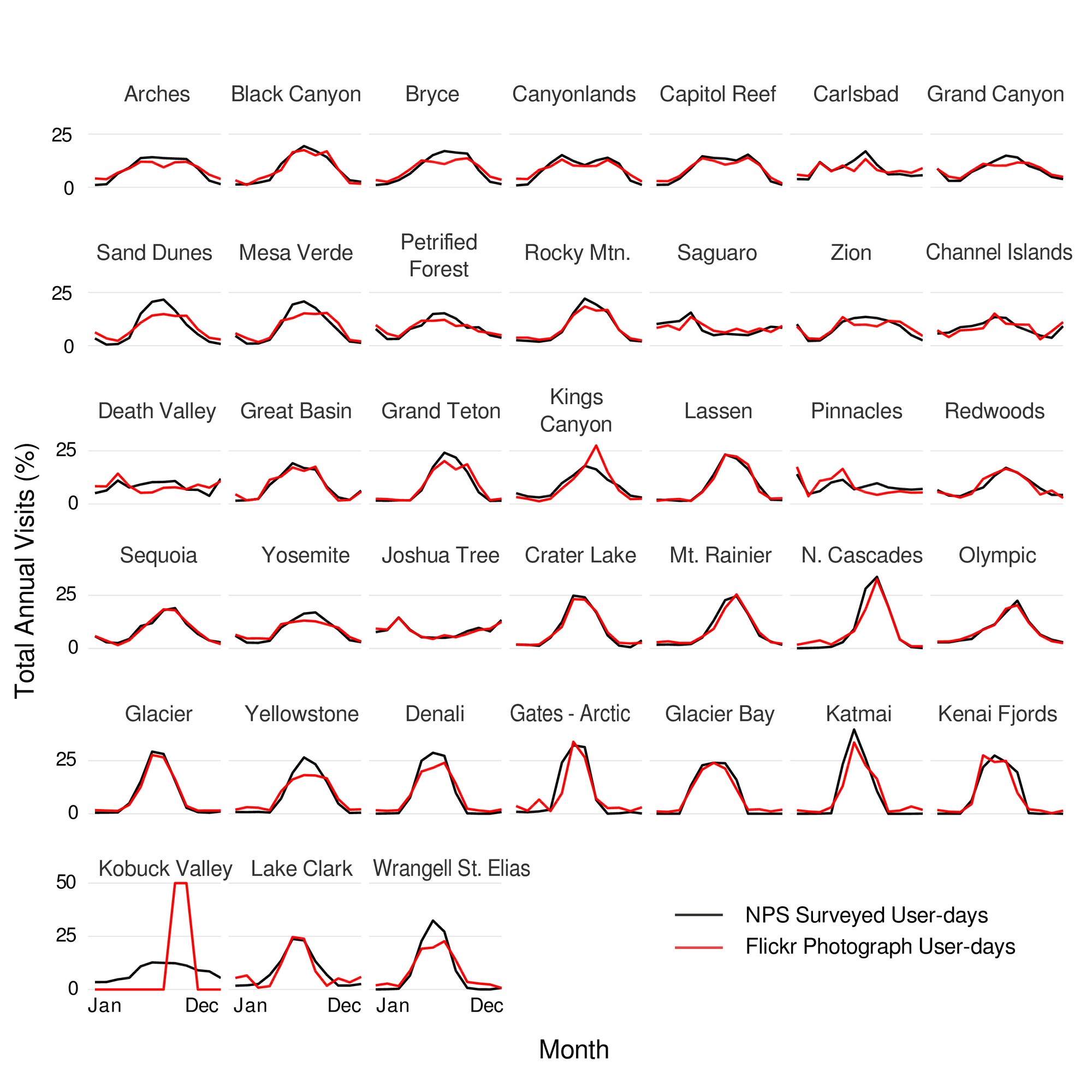
To improve our models and increase their predictive power, we are exploring methods to account for potential biases and issues of representation in social media data. Indeed, certain types of people are more likely to use social media, share their experiences, or geolocate their posts. Thus, our current projects explore differences in results across social media platforms, user groups, and destinations in order to control for issues of representation, in the same ways that traditional intercept surveys address issues of hypothetical and recall bias.